March 6, 2024
New research by Exponent Senior Associate Elif Erkal, Ph.D., shows that when it comes to using machine-learning to predict worker safety on construction sites, measuring success rates is just as valuable as measuring failure rates.
Dr. Erkal, in collaboration with Construction Safety Research Alliance Executive Director Matthew Hallowell and Associate Director of Research Siddharth Bhandari, has published "Predicting Serious Injury and Fatality Exposure Using Machine Learning in Construction Projects" in the Journal of Construction Engineering and Management, Volume 150, Issue 3.
Dr. Erkal's work seeks to address the high, persistent serious injury and fatality (SIF) rates inside the construction industry, which has long been one of the deadliest in the U.S. In 2019 alone, there were 1,102 construction worker fatalities, the highest number of deaths in construction since 2011.
To combat these numbers, the construction industry is turning to machine learning to analyze and predict Serious Injury and Fatality Exposures. Researchers have traditionally used injury and failure data for these models, but they did not provide accurate predictions. Dr. Erkal and her co-authors are not only using failure data, but success data to yield more meaningful and accurate results that can be used to better predict — and therefore help prevent — Serious Injury and Fatality Exposures on construction sites.

"Predicting Serious Injury and Fatality Exposure Using Machine Learning in Construction Projects"
From the publication: "The most influential factors in the models included the crew experience working together, supervisor experience with the crew, total number of workers under the supervisor's purview, and the maturity of leadership development programs for frontline supervisors."
Insights
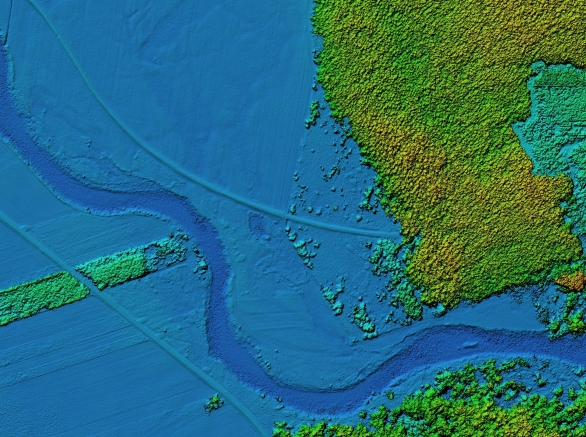