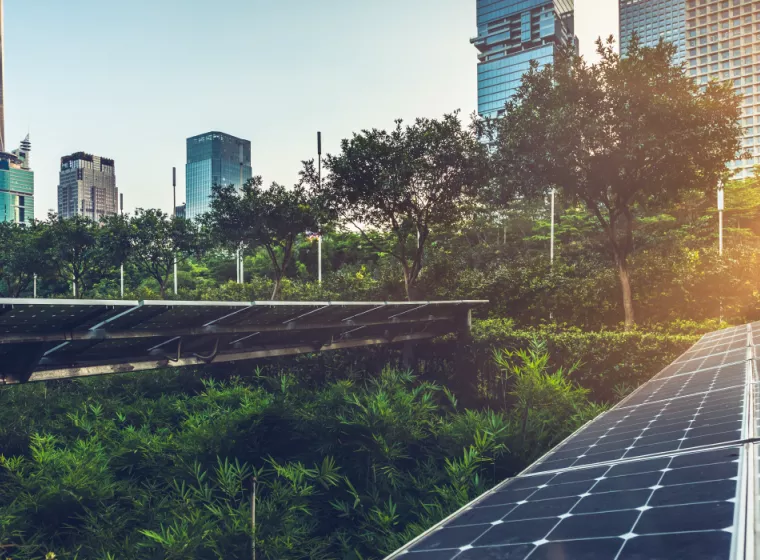
Thought Leadership & Industry Analysis
Facing a complex challenge that requires both industry experience and technical expertise? See how we solve.
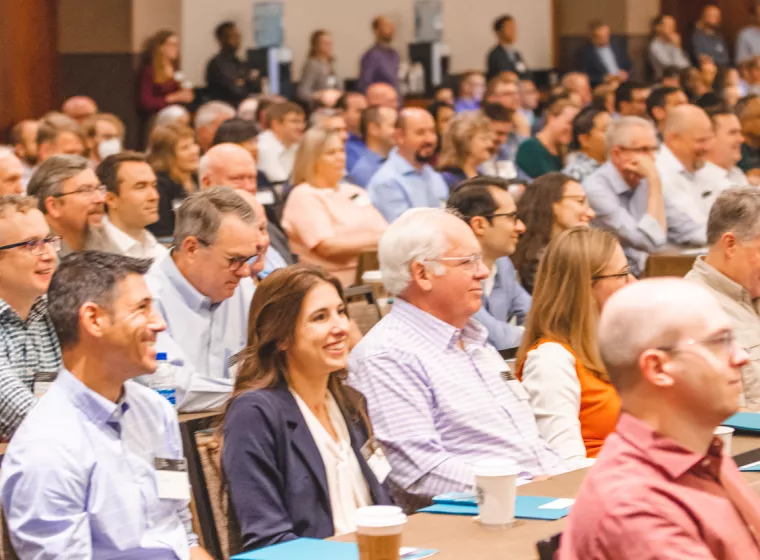
Events & Webinars
Connect with Exponent's multidisciplinary experts at live events and webinars.
![Occupational & Environmental Health Risk Assessment [HS]](/sites/default/files/styles/cards_home_card/public/media/images/GettyImages-949947294.jpg.webp?itok=n2F9dJ4G)
Alerts
What you need to know now across more than a dozen industries.
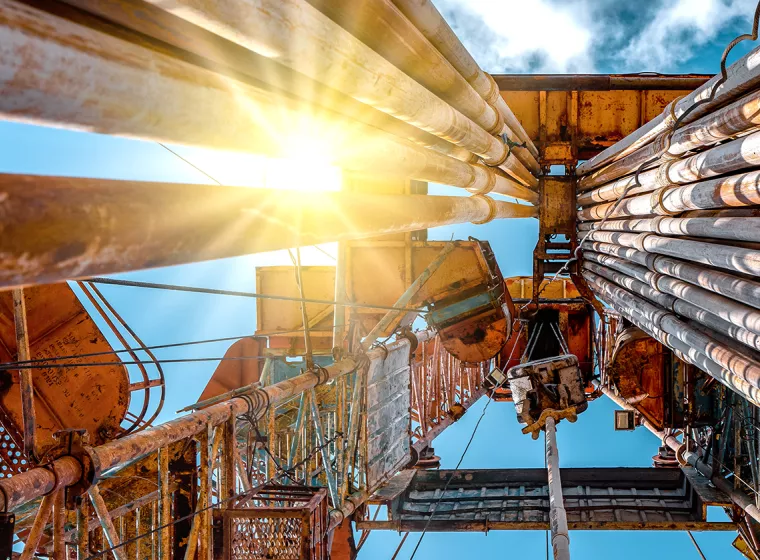
Case Studies
Learn how we apply multidisciplinary thinking to build bespoke solutions for clients in the real world.
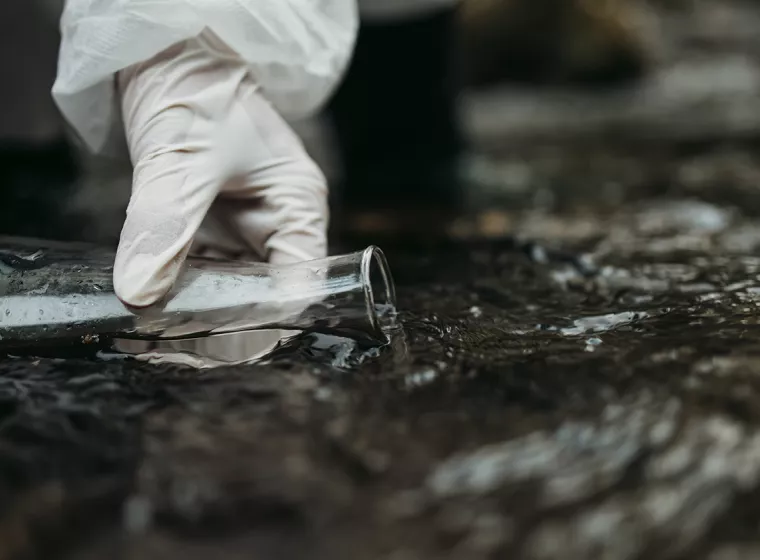
Publications
Read how our original research across 90+ disciplines is advancing engineering and scientific knowledge and standards around the globe
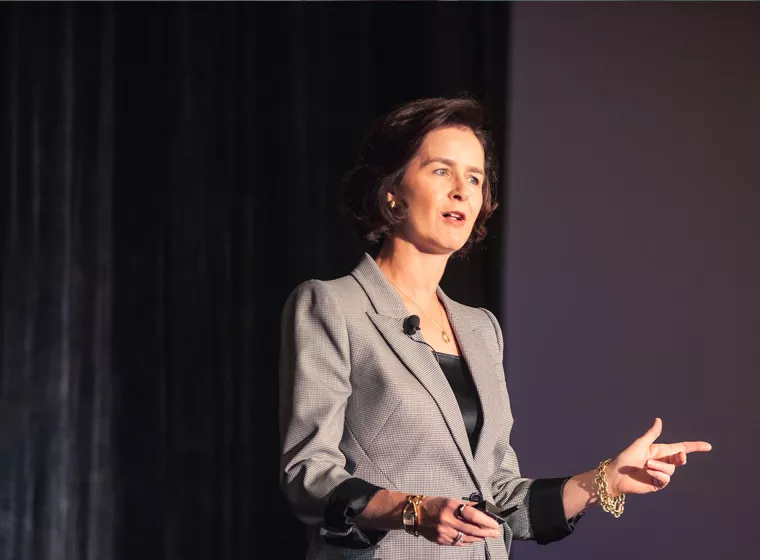
Announcements
Read about Exponent's people, partnerships, and other key firm news.