June 23, 2022
Enterprise data management solutions for climate-challenged utilities
Extreme weather events, once rare a half century ago, affected every region of the United States in 2021 alone. From megadroughts to rapidly intensifying hurricanes, tornado outbreaks, and record-breaking temperatures, our public infrastructure is being stressed to the extremes of its original designs.
The National Oceanic and Atmospheric Administration has been tracking billion-dollar-plus events since 1980 and reports that the past five years account for 34.4% of total weather disaster costs ($742 billion out of nearly $2.2 trillion), underscoring that extreme weather is not only getting more frequent but also more costly.
This fast-evolving landscape has made salient an overlooked aspect of 21st-century reality: our water, gas, electric, and transportation infrastructure is vast, complex, and fragile to an increasingly volatile environment. For utilities, some of the most urgent concerns include avoiding expensive shutdowns; sustaining services under increasingly strained conditions; investing in smarter, more resilient infrastructure; and minimizing liability.
Effective use of data is key to unlocking critical decisions that protect companies, stakeholders, customers, and communities. We know that a good solution involves being data-informed — the question is, how do you get started?
The challenges of off-the-shelf solutions
The primary goals of all utilities in implementing data analytics or management systems are to define and prioritize decisions and actions. To achieve these goals, organizations need up-to-date or real-time data managed through dynamic enterprise-level solutions. These solutions must be capable of absorbing decades of institutional subject matter expertise while keeping pace with skyrocketing demands for better decision-making. Given a market flooded with service providers and software platforms, however, many companies struggle to realize the full potential of what their data can offer. Why is that?
Data is highly specific to the business it comes from. How data is collected, stored, and retrieved reflects a company's history of decision-making, organizational structure, training programs, and personnel management. In contrast, most enterprise data management solutions are driven on the premise that organizational problems are pure data problems that can be solved by data alone. What these technology-based approaches miss is that data models are not mirrors of the physical world or its processes. Data is highly influenced by assumptions, practical limitations, and embedded knowledge that only the subject matter experts who collect and maintain the data understand.
For utilities, these subject matter experts may include mappers, land-use experts, environmental specialists, design engineers, vegetation management, risk and reliability professionals, and construction crews. Each team brings a unique and critical perspective to the table; excluding those perspectives in favor of abstract methods can result in costly errors.
It takes strong partnerships between data specialists, software developers, and subject matter experts to build successful, integrated products that can be deployed through an enterprise data management system.
Data identification, collection & curation
Before building or revamping an enterprise data management program, utilities can start by identifying what decisions need to be made. With at least a few key decisions identified, utilities can set a compass for what data must be collected and curated.
For example, an electric utility would like to identify anomalous mechanical failures of different conductor types based on unusual outage or repair frequencies. Data that will need to be collected for this analysis include a record of historical outages (including the cause category, location, and duration) and repair records (including the work done, the component worked on, and the date completed). The details become even more complex when you consider nuances like how an outage cause is classified (for example, the labels may have changed over time).
High-quality data curation is complex for any organization. Data must be clean and useable (for example, of high enough fidelity or refresh rate to capture physical and operating conditions), robust, and compatible with downstream systems such as risk and reliability models.
For organizations that manage geographically dispersed assets, such as electrical transmission and distribution circuits, pipelines, and railways, accurate data collection is only the first step and depends on gathering multiple data points for thousands of individual system components, including their locations, maintenance and repair histories, inspection reports, in situ testing results, engineering analyses, and environmental data. Curating this data to develop a responsive data ecosystem is one of the biggest challenges utilities must contend with.
Take a water utility with 1,000 miles of pipeline serving one million customers. This utility may have separate departments managing its geographic data (for example, tracking where their buried pipe sections are located, the soil conditions, and proximity to buried gas lines), maintenance records, field inspection data, engineering designs, and customer billing. These departments are all structured as separate entities, but the data they generate must be combined to make accurate enterprise-level decisions. Whereas unintegrated data streams can allow for risk, reliability, and resiliency to be computed at the level of an individual pipe segment, these would not offer visibility into system-level risks or error detection/inconsistencies.
As an example, if the water utility is planning repair work based on recent maintenance records but begins work without considering recent field inspection data or adjacent buried features, the company may not only invest time and money where it isn't needed but create substantial risk around buried gas lines.
Quality analysis, clash detection & repair
Once organizational data is fully integrated, it is critical to cross-check it for inconsistencies and errors. This process is nearly impossible with separate data streams. Whereas partial integration can afford manual opportunities, full integration enables automated capture and analysis for continuous review, correction, and reporting.
However, even utilities that achieve full integration rarely have a process in place to both identify anomalies ("clash detection") and systematically trigger data record repair, due in part to siloed lines of business that don't trace data back to their original sources across many departments and individuals.
In contrast, taking a technical view of how an error surfaced (whether through a construction crew, mapper, design engineer, etc.), what it may represent in the larger data ecosystem, and how it could impact decision-making can help prevent repeat occurrences and avoid erroneous assumptions.
For example, one of Exponent's clients hired teams of contractors to build numerical (finite element) models for tens of thousands of overhead electrical transmission structures and hundreds of overhead circuits. The goal was to integrate the output of these numerical models with other field data to determine the remaining strength capacity of their overhead transmission structures (including all attachments such as insulators, cold end hardware, and conductors). These numerical models were complex and took time to build and validate.
On occasion, by the time a numerical model was finished, the overhead transmission structure had already been replaced in the field — which, if left unchecked, would have carried serious implications for downstream risk modeling. To account for this possibility, Exponent designed a check that was built into the data processing step and executed with every refresh of the risk model, ensuring the numerical models were aligned with the reality in the field and preventing planning and investments from going in the wrong direction, with millions of dollars at stake.
Data analytics & visualization
With a fully integrated, rigorously "clash detected," and systematically repaired data ecosystem in hand, the analytics needed to empower operational and planning decisions can take place. For example, when evaluating the risk and reliability of a network of assets (in this case, consider the wood poles and crossarms with all attachments for an overhead electric distribution system), there are three customizable categories to consider:
Threat: The state of the asset relative to its age and environmental conditions (such as rate of corrosion, decay, or mechanical wear).
Hazard: The exposure of the asset to environmental events, such as wind, earthquakes, flooding, and extreme temperatures.
Consequence: A quantitative measure of the effect of an event, for example, the number of customers that will lose power if a particular electrical transmission structure fails on a particular circuit.
In broad strokes, the output of these models should offer valuable insights into asset resilience, system resistance, and inspection planning. Since risk can vary with environmental conditions on any given day, so too should the models that inform decision-makers.
Think about a transportation agency assessing wildfire risk to a particular community, which depends on the ability of that community to evacuate when a mandatory order is given. Key risk factors include the availability of vehicles, roadway capacity, and coordination with law enforcement to set up evacuation corridors and allow access for emergency response vehicles.
Historical analysis of traffic patterns coupled with population density and vehicle ownership are a few inputs that would be critical to understanding risk — and determining where actions are needed, such as long-term road expansion to promote safe egress or deployment of a mobile application that directs residents to follow specific evacuation routes to coordinate traffic flow and provide accountability of people evacuated.
Act & evolve
Risk- and reliability-driven choices will be prioritized naturally by the level of threat, hazard, and consequence, but they must also be weighed against the feasibility of different choices.
In the transportation agency example, if road expansion is urgently needed on the cusp of wildfire season, the organization will have to decide whether the project can be completed without increasing risk by blocking roads with construction or whether it should be delayed. A strong enterprise data management system can be leveraged not only to make better critical decisions but also to continue evolving. For example, a model that influences behavior may become less predictive as behavior changes, hence it must be adaptable as the system it seeks to measure and predict also changes.
How Exponent Can Help
Exponent has built custom software solutions owned and operated entirely by our clients that can be run at the scale of their data systems. Our comprehensive, bespoke solutions have already been deployed to utility clients and encompass the full range of data needs, including identifying core problems at the required depth of technical data and engineering expertise; leveraging recognized risk and reliability methodologies; collecting and maintaining high-quality data; building validated models, such as risk models; and deploying decision-facilitating software products built on these models through operating systems of each client's choice. We work hand-in-hand with our clients to understand their data management and design software products that will integrate with their existing technology platforms.
Exponent's solutions teams combine subject matter expertise with data science savvy, meaning the people building your solution intimately understand the decisions you need to make. With this approach, Exponent lowers the barrier to taking proof-of-concept ideas and scaling them to production-ready products that clients can use in short time.
Insights
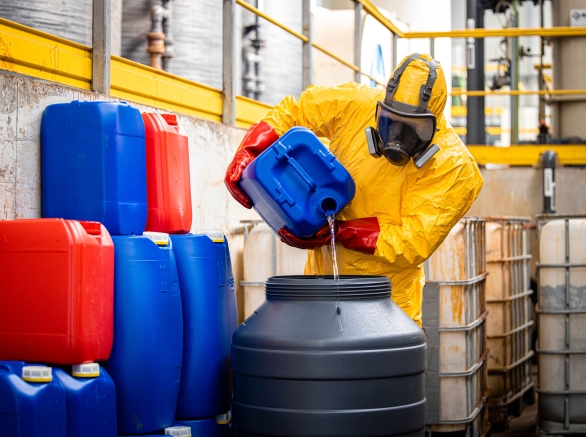