How do Exponent's multidisciplinary data science teams hone solutions for tough data acquisition problems?
For data science solutions, good raw data is imperative to good results. Coordinating data acquisition activities and ensuring collection efforts align with accurate insights takes a seasoned team. Exponent's multidisciplinary data scientists, statisticians, and industry experts assist clients with requirements gathering, roadmap development, data collection, and testing services.
Existing data expertise.
Exponent is highly skilled at extracting information from data warehouses, remote servers, data lakes, on-site servers, and other cloud-based technologies in preparation for new database development or data assessments.
Novel data expertise.
Exponent provides high-quality data sampling and test development services for every size and type of business, including asset analysis, product development studies, human subject testing for healthcare and electronics, and cutting-edge vehicle and transportation studies. When your organization needs to establish proof of concept, meet regulatory requirements, or conduct market analysis, a well-designed study or sample can create cost efficiencies and provide critical insights.
Capabilities
Our scalable solutions save clients time and resources by quickly assessing the project and identifying critical data acquisition and development requirements.
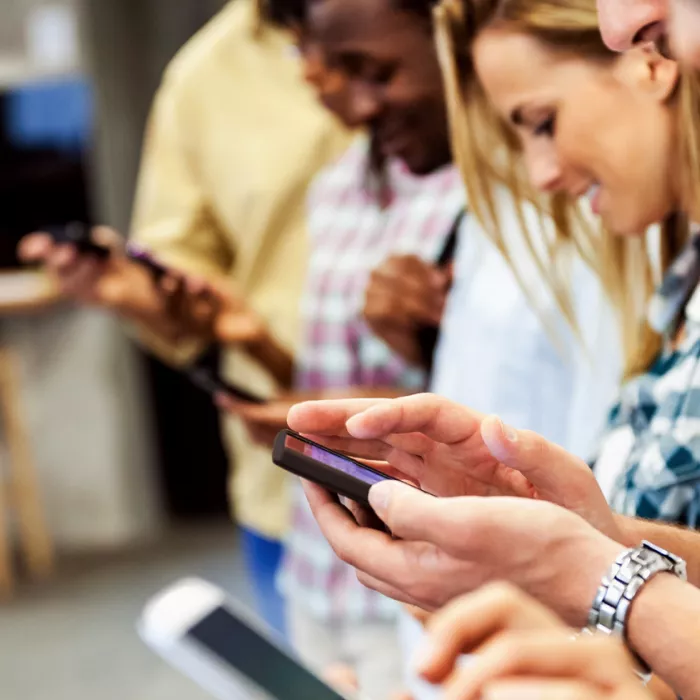
Intentional Data Acquisition
We help you design fit-for-purpose data acquisition projects. Whether you're improving algorithms for biometric data collection or looking to validate a clinical study through real world evidence, Exponent can help you innovate and solve for challenges impacting performance, health equity, diversity and inclusion.
Our Capabilities Are Unparalleled
With expertise in over 90 disciplines and hundreds of capabilities, tools, and methodologies — we get to the root of even the most complex challenges and give you the objective answers you need.
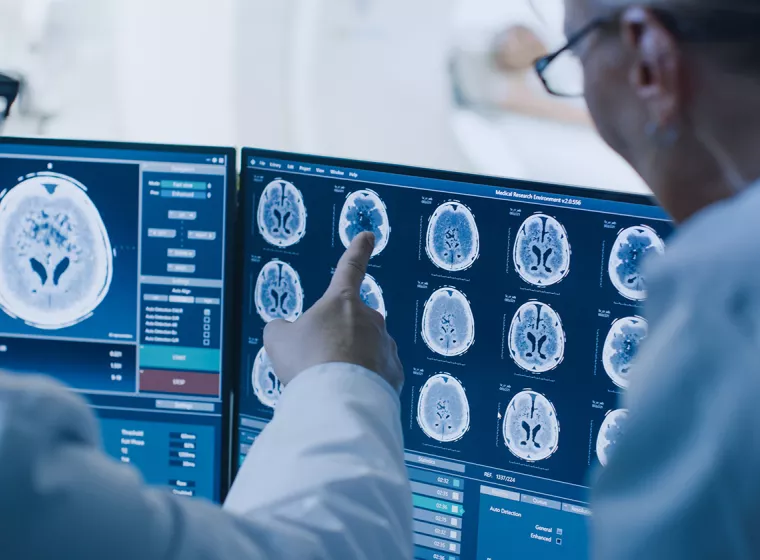
Data Acquisition
Effective data collection from publicly available data, human participants, bespoke testing, surveys, and more.
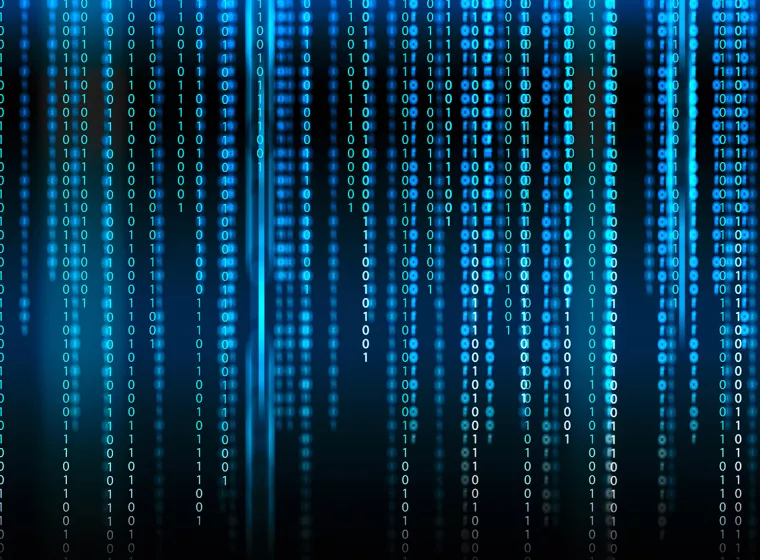
Data Cleaning & Organization
Organize your data to compel results through accurate and insightful intel.
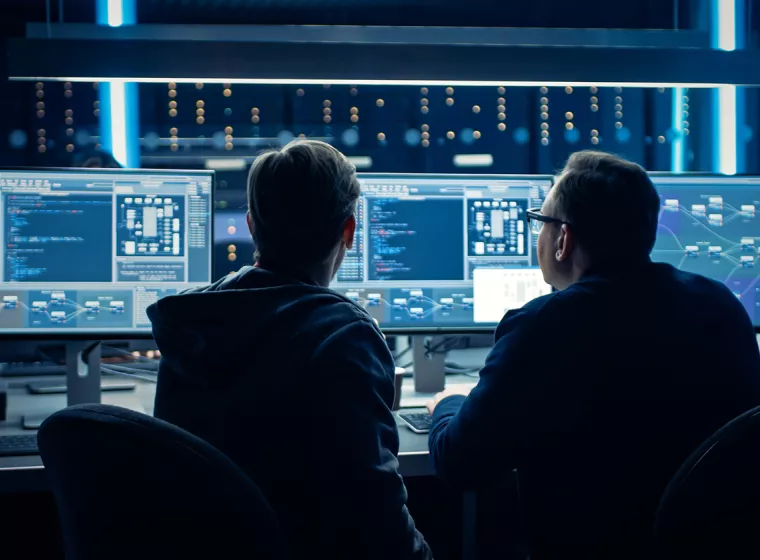
Data Analysis
Strategic guidance leveraging state-of-the-art analytical tools, including statistical modeling, machine learning, artificial intelligence, and more.
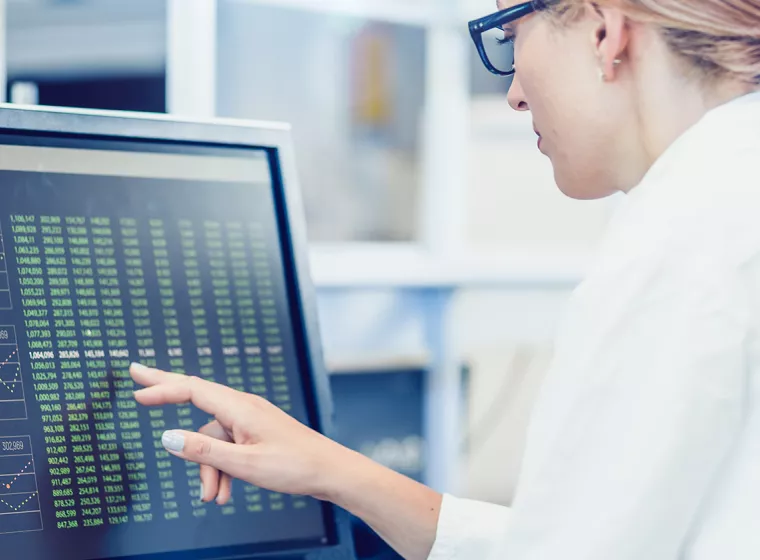
Data Insights: Decide
Data insights for improved decision-making, leveraging risk-prediction models, financial forecasts, cost evaluations, and custom statistical models.