
Are Your Forest Carbon Offsets Going Up in Smoke?
How can Exponent's advanced environmental analytics help you empower decision-making?
Uncovering the root causes of environmental changes and identifying potential risks are central to our environmental modeling and analysis methodologies. Coupled with our advanced analytical and modeling capabilities and decades of experience investigating environmental issues, Exponent is uniquely qualified to consult on the most pressing ecological and environmental challenges.
Our globally recognized ecological and environmental science consultants have experience conducting net environmental benefit analysis (NEBA) for major oil spills, providing compelling models for toxic tort cases and assessing chemical bioavailability in numerous environments. Armed with sophisticated analytics and modeling, our clients can better navigate complicated environmental issues and discover breakthrough insights.
Capabilities
Exponent delivers powerful, evidence-based insights for a clearer path forward with our robust environmental modeling and analysis services.
Our Capabilities Are Unparalleled
With expertise in over 90 disciplines and hundreds of capabilities, tools, and methodologies — we get to the root of even the most complex challenges and give you the objective answers you need.
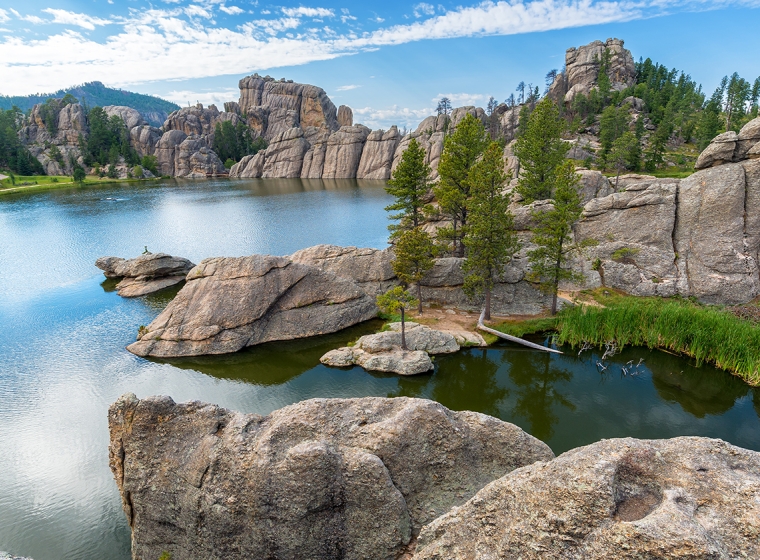
Environmental Permitting
Extensive support for compliance with local, state, and federal permitting requirements.
![[EBS] Ecological & Biological Sciences - Environmental Risk Assessment - grey heron grazing in fresh water](/sites/default/files/styles/cards_home_card/public/media/images/grey-heron-2022-01-04-23-54-37-utc%281%29_0.jpg?itok=9DrVPC-4)
Environmental Risk Assessment
Risk assessments for legacy spills and contamination, potential project and product impacts, and environmental remediation support.
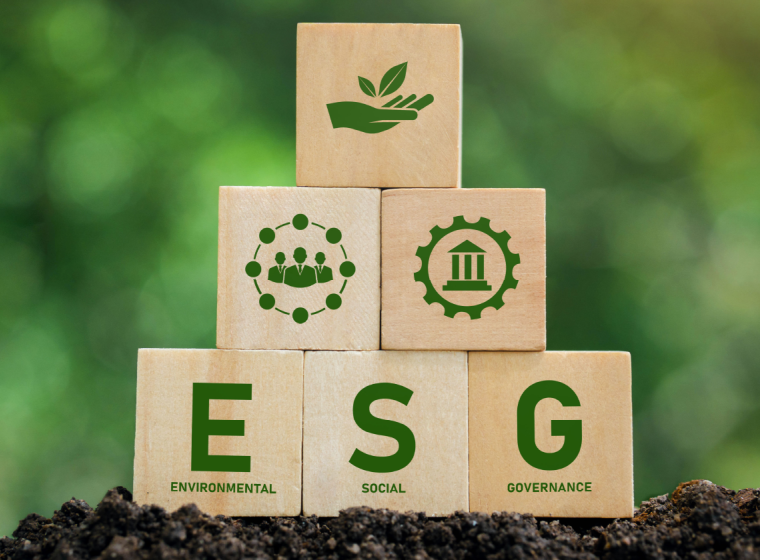
Sustainability
Meet changing sustainability regulations with rigorous science and experienced consulting services.
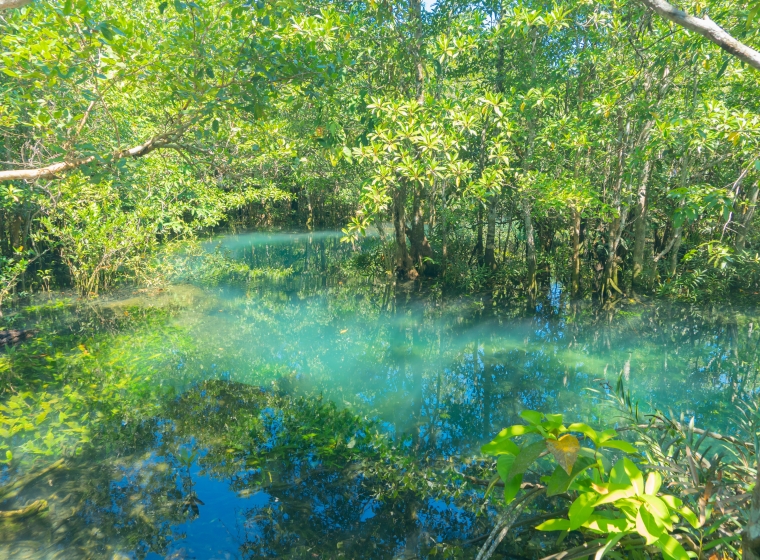
Natural Resource Damage Assessment
Multidisciplinary natural resource damage assessments (NRDAs) to support navigating complex interactions with federal, state, and tribal trustees.
Experts
Our global and comprehensive expertise across industries gives us a deep understanding of current challenges, best industry practices, and the implications of emerging technologies.
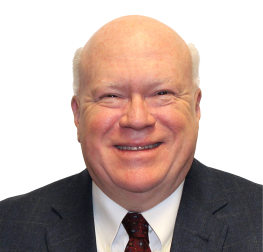
Practice Director and Principal Scientist
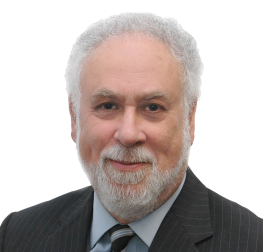
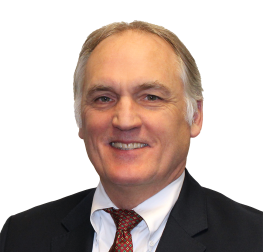
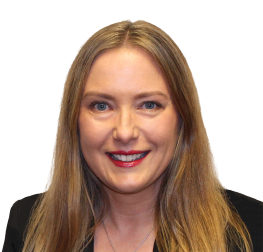
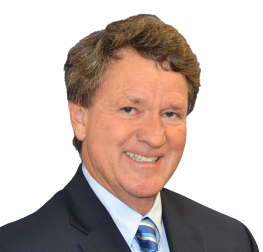
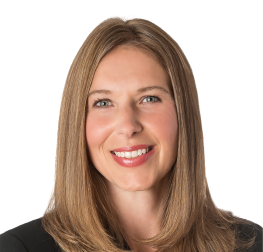
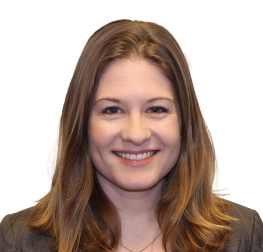
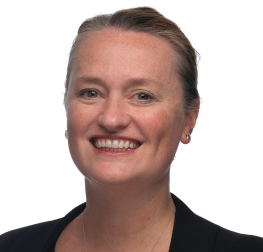
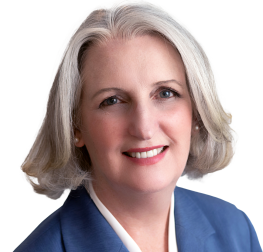
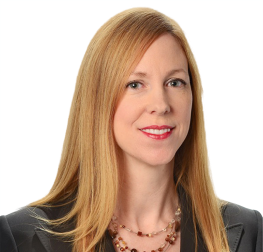
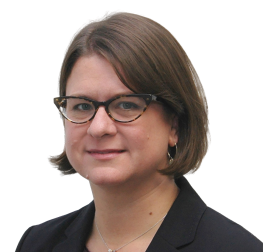
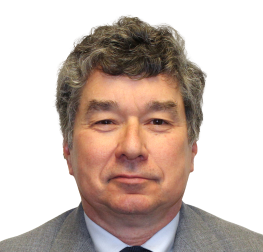
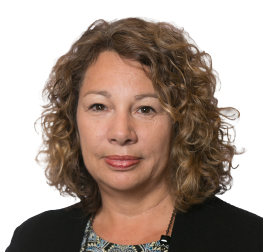

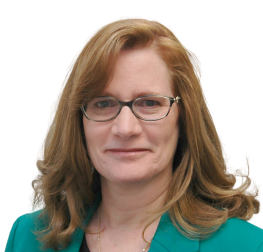
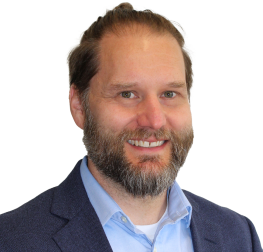
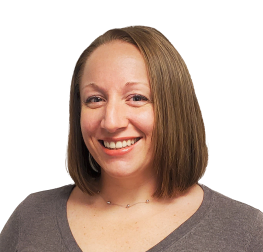
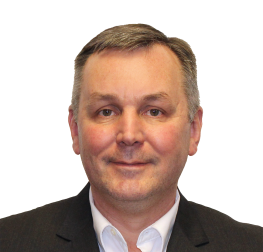
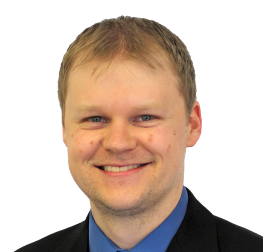
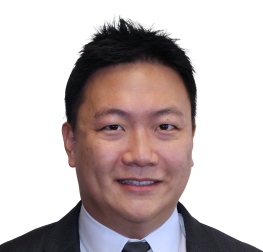
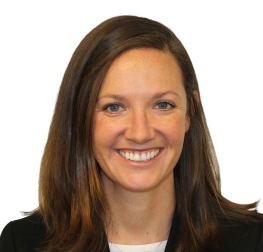

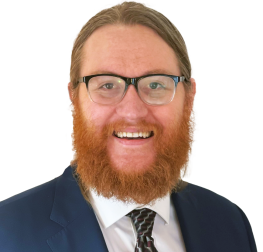

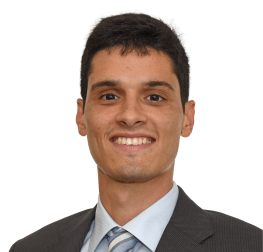
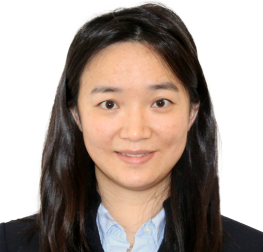
Insights
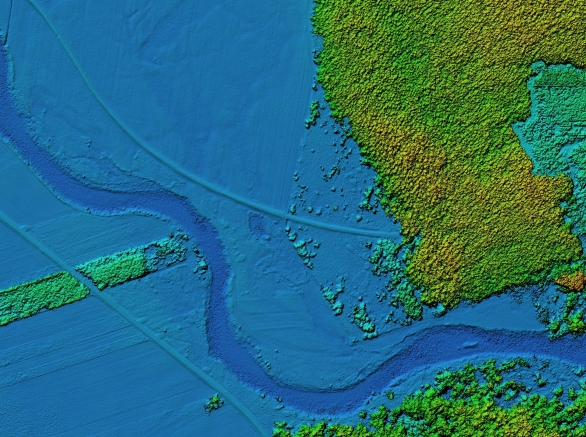